
How AI & Advanced Analytics Transforming RTSM/IRT Systems
In the rapidly evolving landscape of clinical trials, technology plays a pivotal role in ensuring efficiency, accuracy, and compliance. Among the critical components of clinical trial management are RTSM (Randomization and Trial Supply Management) and IRT (Interactive Response Technology) systems. These systems manage patient randomization, drug supply logistics, and real-time trial data handling. Traditionally, RTSM/IRT systems have relied on rule-based algorithms and manual oversight, which, while effective, have limitations in scalability and adaptability.
Today, the integration of Artificial Intelligence (AI) and Advanced Analytics is revolutionizing these systems, enabling smarter, faster, and more adaptive clinical trial management. This blog delves into how AI and advanced analytics are transforming RTSM/IRT systems, the benefits they bring, challenges to adoption, and the future outlook of this technology-driven evolution.
Understanding RTSM and IRT Systems in Clinical Trials
Before exploring the impact of AI, it’s essential to understand the foundational role of RTSM and IRT systems:
- RTSM (Randomization and Trial Supply Management): This system controls patient randomization to treatment arms and manages clinical trial supplies, ensuring the right drug is delivered to the right patient at the right time.
- IRT (Interactive Response Technology): IRT systems enable real-time interaction via telephone or web-based portals for patient enrollment, randomization, dosing, and drug inventory management.
Both systems are vital for maintaining trial integrity, managing complex logistics, and ensuring compliance with regulatory standards.
The Role of AI and Advanced Analytics in RTSM/IRT Systems
AI and advanced analytics bring a new dimension to RTSM/IRT systems by leveraging machine learning, predictive modeling, and data-driven decision-making. Here are some key areas where AI is making a difference:
1. Intelligent Patient Randomization
Traditional randomization methods often rely on fixed algorithms that may not account for dynamic patient characteristics or site performance. AI-powered randomization can:
- Analyze real-time patient data to optimize treatment arm assignments.
- Balance patient demographics and baseline characteristics more effectively.
- Adapt randomization strategies based on ongoing trial data to reduce bias and improve statistical power.
2. Predictive Supply Chain Management
Managing clinical trial supplies is complex due to unpredictable enrollment rates and patient dropouts. AI-driven analytics can:
- Forecast drug demand more accurately by analyzing historical and real-time trial data.
- Optimize inventory levels at trial sites to prevent shortages or overstock.
- Predict potential supply chain disruptions and suggest mitigation strategies.
3. Enhanced Patient Enrollment and Retention
AI algorithms can analyze patient recruitment patterns and site performance to:
- Identify high-performing sites and patient populations.
- Predict enrollment bottlenecks and recommend targeted interventions.
- Improve patient retention by identifying risk factors for dropout early.
4. Real-Time Monitoring and Risk-Based Management
Advanced analytics enable continuous monitoring of trial metrics, allowing:
- Early detection of anomalies or protocol deviations.
- Dynamic risk assessment to prioritize monitoring resources.
- Automated alerts and decision support for trial managers.
5. Automated Compliance and Audit Trails
AI can enhance regulatory compliance by:
- Automatically generating detailed audit trails.
- Ensuring data integrity through anomaly detection.
- Streamlining documentation for regulatory submissions.
Benefits of AI and Advanced Analytics in RTSM/IRT Systems
Increased Efficiency and Speed
AI automates complex tasks such as randomization and supply forecasting, reducing manual workload and accelerating trial timelines.
Improved Accuracy and Data Quality
Machine learning algorithms minimize human error and enhance the precision of randomization and supply management processes.
Cost Savings
Optimized supply chains and improved patient recruitment reduce wastage and operational costs.
Enhanced Patient Safety and Trial Integrity
Adaptive randomization and real-time monitoring help maintain balanced study arms and quickly identify safety concerns.
Scalability and Flexibility
AI-powered systems can handle increasing trial complexity and adapt to evolving protocols without extensive reprogramming.
Challenges in Implementing AI and Advanced Analytics in RTSM/IRT
Despite the clear advantages, several challenges must be addressed:
- Data Privacy and Security: Handling sensitive patient data requires robust cybersecurity and compliance with regulations like GDPR and HIPAA.
- Integration with Legacy Systems: Many clinical trial organizations operate legacy RTSM/IRT platforms that may not seamlessly integrate with AI tools.
- Regulatory Acceptance: Regulatory agencies require transparency and validation of AI algorithms, which can be complex.
- Change Management: Training staff and shifting organizational culture to adopt AI-driven processes takes time and effort.
- Data Quality: AI’s effectiveness depends on high-quality, comprehensive data inputs, which may not always be available.
Best Practices for Successful AI Integration in RTSM/IRT Systems
1. Start with Clear Objectives
Define specific goals such as improving randomization balance or optimizing supply forecasts to guide AI implementation.
2. Ensure Data Governance and Compliance
Establish strong data governance frameworks and ensure compliance with relevant data protection regulations.
3. Choose Scalable and Interoperable Solutions
Select AI platforms that can integrate with existing RTSM/IRT systems and scale with trial complexity.
4. Validate AI Models Thoroughly
Conduct rigorous validation and testing of AI algorithms to meet regulatory standards and build stakeholder confidence.
5. Invest in Training and Change Management
Educate clinical trial teams on AI capabilities and workflows to facilitate smooth adoption.
The Future of RTSM/IRT Systems with AI and Advanced Analytics
The future holds exciting possibilities as AI and analytics continue to evolve:
- Integration with Wearables and IoT: Real-time patient data from connected devices will feed directly into RTSM/IRT systems for dynamic trial management.
- Blockchain for Transparency: Combining AI with blockchain could enhance data security and auditability.
- Adaptive Trials: AI will enable highly adaptive trial designs with continuous learning and optimization.
- Personalized Medicine: AI-driven randomization could tailor treatments based on individual patient profiles, improving outcomes.
Conclusion
AI and advanced analytics are transforming RTSM/IRT systems from static, rule-based tools into intelligent, adaptive platforms that enhance clinical trial efficiency, accuracy, and compliance. By harnessing these technologies, sponsors and CROs can better manage patient randomization, optimize supply chains, and monitor trials in real-time, ultimately accelerating the development of safe and effective medical treatments.
Embracing AI in RTSM/IRT systems is no longer optional but a strategic imperative for organizations aiming to stay competitive and deliver high-quality clinical trials in today’s data-driven healthcare environment.
If you’re interested in exploring AI-powered RTSM/IRT solutions or need guidance on integrating advanced analytics into your clinical trial operations, feel free to reach out to DDi for expert support.
Get the latest updates from DDi
Explore Topics
- Automation & AI (5)
- Clinical Automation (8)
- Consumer Health (1)
- IRT & Clinical Supplies (21)
- Labeling (16)
- Regulations (23)
- Regulatory Automation (14)
- Regulatory Biopharma (2)
- Regulatory Content Management (5)
- Regulatory Information Management (21)
- UDI (11)
- Writing (12)
Recent Blogs
Digital RIM for Next-Gen Medic…
Regulatory Information ManagementHow Automation is Transforming…
Automation & AIWhy eIFU is Future of Device L…
Regulations
Previous Post
Next Post
Related Posts
CONNECT WITH US
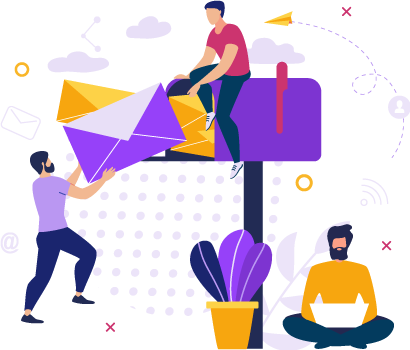
Let's talk about how DDi can help you